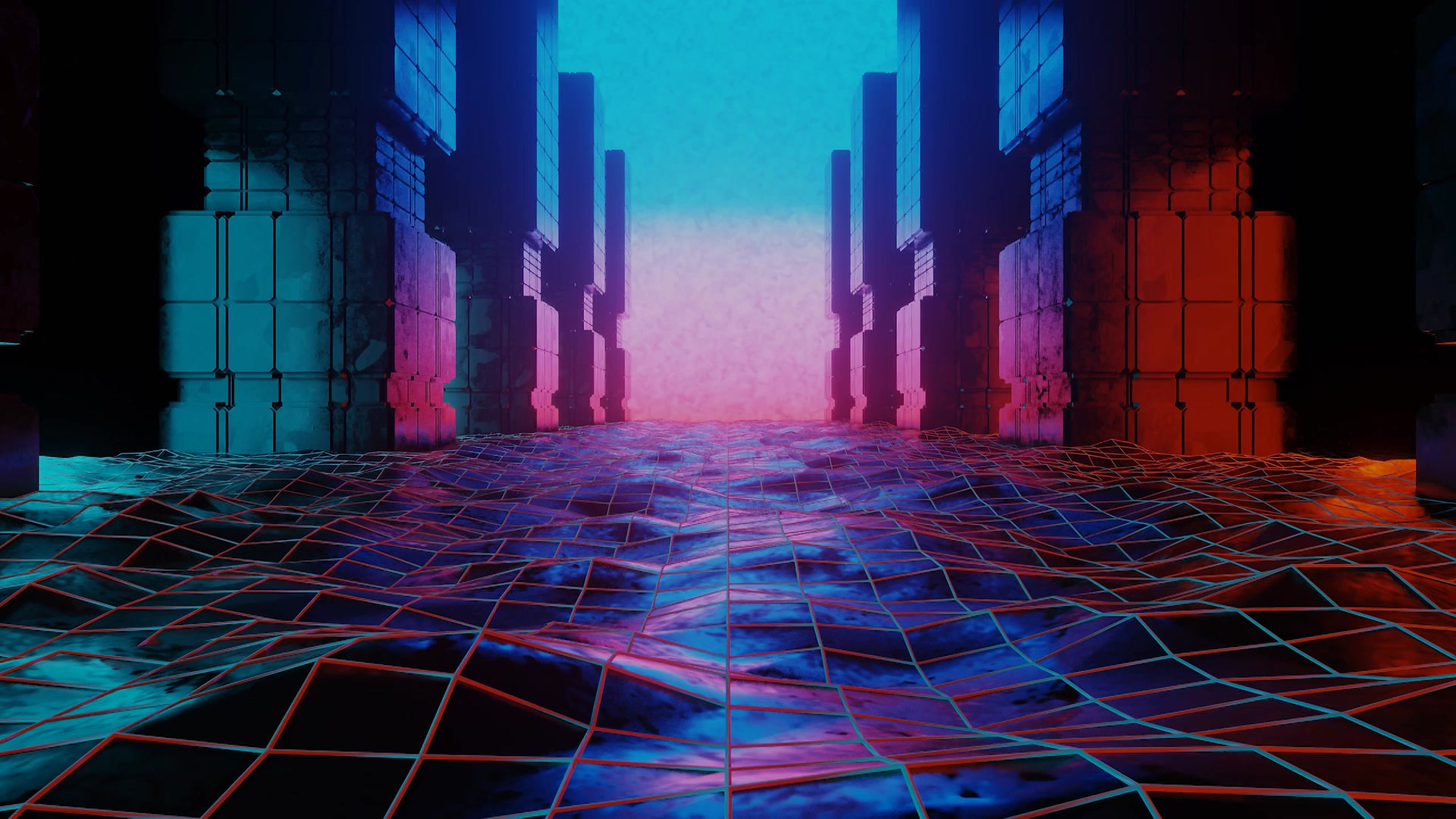
Search Results
40 items found for ""
- The Future of Data Quality Solutions: Management Criteria and Regulatory Compliance
Data quality is a business priority. Every day your business gathers data, including confidential and sensitive information regarding customers, target markets, internal finances, and more. With continually evolving privacy and compliance regulations, such as CCPA (California Consumer Privacy Act), CPRA (California Privacy Rights Act) and GDPR (General Data Protection Regulation), maintaining regulatory standards is necessary for avoiding fines, but it also helps you protect your business and retain your customer trust. Is your business prepared to prevent potentially devastating complications that could wipe out significant revenue? Low-quality data is a disaster waiting to happen, and the best time to determine your plan is before disaster strikes. Consequences of low-quality data The consequences of low-quality data include: Diminished reputation. Fines for inaccurate or late reporting to regulatory bodies. Inferior decision-making. Loss of revenue. And that’s not all. It costs employee time to investigate, identify, and correct problems, and in the worst cases, even equipment and inventory can be casualties. Regulatory compliance The most obvious consequence of noncompliance is the regulatory fines your company can incur. Data quality is required to meet standards for privacy, security, accuracy, reporting, and shareability. Did you know, for example, that GDPR requires businesses to correct inaccurate or incomplete personal data? But there are benefits to complying with data quality rules and regulations besides avoiding fines. Adhering to regulations helps you ensure overall data quality, which leads to higher efficiency, a streamlined customer experience, and increased revenue over time. Following regulations establishes the minimum baseline for your data quality. From there, you can improve upon your data quality until it reflects your desired management criteria. Getting ahead of regulatory compliance, rather than letting it catch you off guard, can be the difference between floundering and flourishing. Data quality management criteria Analyzing your data quality needs, and the risks associated with neglecting them, allows you to create the best management criteria for maintaining and optimizing your company’s data quality. General management criteria include: Accuracy. One of the primary factors for data quality management is data accuracy. Data that doesn’t accurately reflect its source is useless at best and catastrophic at worst. Inaccurate data takes time and effort to track and correct. So, protocols for regularly updating data and verifying its accuracy are an essential piece of your management criteria puzzle. Consistency. When gathering data, it should be internally consistent regarding what is recorded and how. Standardize forms, field rules, and use consistent formats and formulas for dates, measurements, and other information. You should also ensure data is consistent with reality. If a record shows an employee’s birth year occurring after the year they were hired, for instance, it is inconsistent with reality. Duplication. Duplicate records occur when data is collected from multiple sources. Duplicates get a bad rap, but it’s important to identify and evaluate them carefully to determine and optimize their value. Completeness. Empty fields in data sets and/or missing data sets are examples of completion issues that should be addressed in your company’s data quality management criteria. Formal, standardized data quality management is necessary for regulatory compliance regarding data privacy and security. Data quality management solution Unaddressed data quality issues can quickly get expensive, whether in regulatory fines, inefficient business activity, poor business decisions, or all of the above. Data management criteria and compliance regulations head off potential data issues in your business. But a total data quality management solution — designed to meet the unique needs of your company — organizes and optimizes your data to correct existing quality issues and avoid future problems. Learn more about total data quality management solutions for your business at xcelerated.com.
- Everything you wanted to know about data lists -- but were too afraid to ask
Private Equity Funcast Jim Milbery and Devin Mathews. Hosted by Devin Mathews and Jim Milbery, partners at ParkerGale Capital, a middle-market private equity group. This bi-weekly show is a lively discussion of the uses of technology to improve business operations for companies with less than $100 million in revenue. "In this week's episode, we are talking with Pam Lang, CEO of Xcelerated Data, a company that focuses on fixing data quality problems leading to better decisions in your marketing organization. Pam explains compiled lists, hotlists, the differences between consumer lists and business lists, and SIC codes versus NAICS codes." Click image above to listen to the episode
- Data Quality Is Critical for Better Business Decisions
Every decision you make relies on some form of data, and the appropriate level of data quality is fundamental to sound business decisions. What can you do to ensure the accuracy of your company’s data in support of well-informed business decisions? Begin with a basic understanding of the significance of data quality. The importance of data quality Data is essential to every business in every industry. Data drives your most expensive activities and your most critical business decisions – from launching a new project to altering your business model. Quality data increases the accuracy of your predictions to allow for better market forecasting and budget planning — and a more positive customer experience. What impact does low-quality data have on your company’s: Reputation? Employee Satisfaction? Project Success? Financial Results? Decision-making, company reputation, and your customers’ experience can all suffer from poor data quality. Measuring data quality Data quality has several metrics by which it can be measured and maintained. These include: Consistency. Are your data sets consistent with one another? Is all your data current, measured objectively, coherent, and reasonable? Imagine losing a $125 million dollar space probe because of something as basic as feet versus meters. If it can happen at NASA, it can happen to you. Accuracy. Is your data correct and representative of the object or reference information? For example, what happens to the reputation of a charity that sends five identical mail pieces to a long-time donor? Inaccurate data can cause anything from a minor inconvenience to a catastrophic failure depending on the context, so ensuring data accuracy is critical. Completeness. Are all your records complete across data sets? Are any records missing relevant information, such as a previous surname or current contact information? While there are other important metrics to consider, these three basics are easy to check in an initial examination of data quality. Problems in any one of these areas can have a significant impact on your business. Improving data quality According to a poll of data professionals, 75% of respondents named data quality as one of their top priorities. So, the next question is: How do you improve your company’s data quality? Recognizing the existence of a problem is the first — and often most important — step to data quality improvement, but without understanding the underlying causes and implementing action to correct the problem, the potential consequences of low-quality data remain. There are specific steps you can take to manage your data quality. Establish data governance. Develop an understanding of your data and how it is used, establish rules for managing how data is collected and/or input, and implement formal, regular data quality maintenance procedures. Perform frequent data checks. Changes in names, addresses, titles, positions, management requirements, or local, state, or federal regulations can all lead to outdated, inaccurate, and incomplete data. Using external validation processes and running appropriate reports, for example, will allow you to catch issues before they grow into serious problems. Incorporate a total data quality management solution. Complex data quality issues require more than a few tweaks. Frequent or persistent problems require a complete solution to resolve current issues and ensure data quality going forward. Implement continuous improvement strategies. It’s unlikely you’ll have data that never changes. With fluctuations in customer information, market trends, and other changes associated with running a business, maintaining data quality is a constant process. When your company’s data quality is low, it impedes your ability to make sound business decisions. Maintaining quality data requires consistent, ongoing, and well-defined processes — a total data quality management solution. Data drives your most expensive activities and your most critical business decisions. Learn more about custom and complete data quality management solutions for your business at xcelerated.com.
- EXPERT Tips for DATA QUALITY: Dreaded Duplicates - kill? keep? combine?
How do you treat your duplicates? Stop for a moment and think about this... The natural reaction for most companies is to delete duplicate records without much thought. But we LOVE duplicates! ..so much information to glean. So, there are really 3 main choices to consider. These choices are not mutually exclusive and when used in combination produce favorable results: Kill - One of the top reasons for deleting duplicates is AFTER the combining of the best fields (see #3). Another reason is that the information in each field is exactly the same. If and when you DO choose to outright delete duplicates - be sure you are deleting the CORRECT records based on a PRIORITY of the best fields! Then - and this is key - keep those duplicates and compare them to your final file to ensure you are removing or keeping the correct records. Keep - There are many reasons and methodologies for keeping duplicates. Duplicates can be an indication of a customer visiting multiple store locations, or completing online and offline transactions. There may be times where you need to maintain historical information such as previous addresses and prefer to keep duplicates but move to a separate file. Very often, data is originating from different systems and it is not feasible to dedupe between these systems, instead, code your duplicates so you can identify and handle them correctly. Combine - Extracting the BEST data points from each duplicate and combining them into one record is one ideal solution. A few examples are cases when there is additional contact information such as phones, emails or digital identifiers, also notes, purchases, and dates of contact. Combining duplicates can occur in many different configurations that bring great power and insight into your data. With a complete Data Quality Management Program, there are some thoughts to consider first BEFORE embarking on any deduping. The details are shared in a previous video that we created but as a quick overview, these thoughts include: What are the inputs/sources of the data? What do you consider a duplicate? (Do you even know? ) and, How will you use the data? Not sure what is the best for your data? Email us and unload your issues! pam@xcelerated.com
- What you need to know about your data
Data — and data quality — are essential to business success in a data-driven world. You don’t need to be a data analyst or a data quality expert to benefit from a complete data quality management solution. But you do need expert assistance. To manage the data quality of your data-driven business, reach out to us.
- What can Xcelerated do for you?
How do you know you need help with your data? What if you have a unique or special data project? Would you know where to go? Aaron Sevigny, President and CEO of Acadia Wealth Management, shares his Xcelerated Data experience.
- Data Definitions
Let's talk definitions / Let's clarify some important terms Data Hygiene / Data Cleansing: Typically a disparate set of standardized processes, such as NCOA or Basic Deduping. Accepts a standard/required input and returns a static set of fields. Processes are typically rigid and do not accommodate the variety of data sources, inputs, and goals. Each step is done independently of the others and priced separately, making it difficult to understand the final cost. Often times laborious reformatting is required. Data Enhancement / Data Enrichment / Data Append: Matching input data to a large standard file consisting of consumers or businesses to add a variety of additional data points. Examples of added data include: Consumers - Demographics, such as Age, Income, Occupation; Psychographics, such as buying behavior; Contact information such is emails and phones. Business - Firmographics such as Revenue, employee size, Industry Codes, etc... Contact information such as emails and phones. Data Quality Solutions: Comprehensive end-to-end process to solve various data issues as a whole. Considers the input data sources, the goals, and desired output to create a unique customized and automated solution. Data quality tools: Tools that are installed in-house or software as a service. They are used to load your data for a wide range of standardization, reporting business intelligence, deduping, combining, etc... Typically they are higher priced and also require the expertise of the business needs and goals end of the data itself. These tools still require a large investment of time and learning. Master Data Management/MDM: is a technology-enabled discipline in which business and IT work together to maintain all aspects of the quality, consistency, and accountability of the data across the entire company. MDM supports the business initiatives and objectives. First-Party Data: First-party data collection is consent-driven and inherently more transparent than other collection methods, and advertisers who can act on collected data will have a leg up against competitors and the fast-changing industry. This will typically include your customer list people that have inquired and provided their information people that have opted in to receive newsletters and shared their information.
- Data Hygiene vs. Data Quality Solutions: What’s the Difference?
The terms “data hygiene” and “data quality” are both well-known and oft used in Big Data circles, but how interchangeable are they? What is the difference between hygiene and quality as applied to stored information? Understanding the language of data maintenance is essential for ensuring your company’s most valuable asset — your business data — is accurate, reliable, and complete. Data hygiene Data hygiene refers to the disparate steps your company employs to keep data accurate and up to date. This is typically accomplished through a collection of rigid processes for standardizing data sets, removing unnecessary or outdated data, and organizing it for use throughout your business. While data hygiene can help to optimize productivity and informed decision-making, it can easily go wrong — with disastrous results up to and including the loss of critical data. Data hygiene processes must be done with careful attention to best practices. Despite the potential risks, data hygiene can be helpful for avoiding redundancy, improving data accuracy, and updating data sets. But it’s only a subset of data quality management for accurate — and useful — data. Data hygiene is a good start, but where do you go from there? Data quality solutions Gartner defines data quality solutions as “the processes and technologies for identifying, understanding and correcting flaws in data that support effective data and analytics governance across operational business processes and decision making.” Data quality solutions are complete, end-to-end, customized processes for solving a variety of data problems in one go. Ensuring data quality is more comprehensive than simply cleaning up redundant data and keeping what you need updated. Data quality solutions encompass the full range of data management processes in one neat package from one vendor partner. Instead of using different, laborious processes for solving data problems, these solutions are customized to fix quality issues and ensure ongoing data accuracy and consistency. Custom solutions are based on data sources, industry priorities, and individual company goals, and their interconnectivity allows you to unify information across different teams and divisions of your business to ensure decision-makers at every level are working from the same — accurate and reliable — data. Data quality solutions simplify day-to-day processing and the business operations that incorporate data. Why it matters Data hygiene is a subset of data quality, but pursuing it without considering the potential consequences of disparate processes invites problems. Disparate processes can miss data issues — and even create a few new ones. Redundant processes waste time and money and frequently fall short of your intended goals — not to mention the problems that can arise from a lack of proper management. Data quality solutions cover more than data hygiene. They are complete solutions to your company’s data challenges, and they continue to safeguard your data against new issues that arise over time. This comprehensive approach to data management improves your valuable data and establishes a custom framework for ongoing, consistent, and efficient processing. In short, data hygiene processes are only a part of improving your data. They are useful on their own when managed carefully, but data quality solutions improve data — quickly and efficiently — for better business decision-making. For a comprehensive answer to your company’s data challenges, data quality solutions beat standalone data hygiene techniques every time. Contact the data experts at Xcelerated Data today to talk about data quality solutions for your business, or sign up to take the database challenge for free. We will find problems in your data you didn't even know existed.
- Custom Data Project for Wealth and Investment Company
Comprehensive financial planning Advanced Strategies The Challenge: To validate a unique stock option investment strategy that maintains high returns while reducing risk. Our Task: Analyze over 1,300 spreadsheets, containing 18 million records of stock-option data that spanned 16 years using a variety of complex parameters including: Trading Dates Expiration Dates Strike Prices Daily Closing Prices ATM (At The Money) & OTM (Out of The Money) Options The Result: Boiled down 18 million records into 3 data sets of about 20,000 records each so our client could verify their theory and create better portfolios for their clients.
- A single vendor solution to your data management problems
Disparate data quality management processes contribute to confusion and in some cases, complete chaos. A single vendor can leverage data quality expertise to manage your business data in a consolidated, end-to-end process that accounts for its source, purpose, and any potential problems. A single source data quality solution increases the accuracy and utility of your company’s data.
- Dirty Data Cleanup
Your new marketing campaign just fell short, despite spending many hours designing, researching and planning. The client is unhappy, even though it was their data. This happens all the time, why? Not having a clear understanding of data processing will destroy your marketing results. For a quick and easy turn around, Dirty Data Cleanup™ is our standard automated process for a "one-size-fits-most" data quality solution.
- The fourth component of the PPT equation
The “people, process, and technology” (PPT) framework helps businesses identify the necessary components for successful change management. Data has long been lumped in with technology, but that’s changing as more companies recognize the fundamental nature of data and the role it plays in their success. Data is finally getting its due. Is your company’s data ready for the spotlight?